Towards a Personalized Physical Intervention Recommender System for Parkinson’s Disease
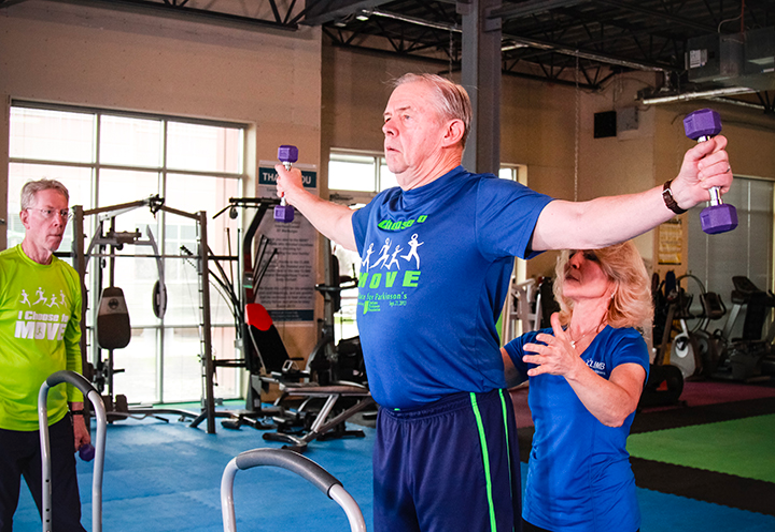
Neurodegenerative conditions, such as Parkinson’s Disease (PD), affect regions in the brain that play a key role in cognitive and motor function, leading to impairments of movement, speech production, memory, reasoning, planning, reflexes, among others. Assessment of disease state is essential in both clinical practice and research in order to measure disease severity, disease progression, and treatment effectiveness. Currently, the clinical assessment of PD relies primarily on subjective evaluations, such as rater-based interviews and questionnaires and tests such as simple rhythmic activities to evaluate motor symptoms and tremor. With the wealth of new mobile technologies, their sensor capabilities, and the resulting data, there are new opportunities to develop digital biomarkers that translate these new data sources into informative, actionable insights. This work utilizes advances in sensing, analytics, and machine learning to design integrated function-specific biomarkers that can provide fine-grained insights into a patient’s disease course for various neurological functions such as cognitive skills, fine and gross motor functions, speech/language, etc. This work also uses the newly developed biomarkers to investigate the effects of various intervention types on functional performance.